Blog
22-01-2024
Long Live AI
The following article comes from the relevance of the considered and flexible application of AI systems in our current work models and the personal experience at two recent conferences about the future of AI- "AI vs Art and Design”, the APDSI Artificial Intelligence and Art Conference (opportunity through .PT) and "Industry Sessions: AI Drives Design ” from EDIT.
We are currently witnessing a divided industry between the faith in Artificial Intelligence as a new fundamental tool for professional practice and the other side that does not recognize AI data and results as trustworthy. The progressive integration of AI into business has proven to be the new trend and according to many for good reasons. We currently live in a growing phase of the implementation of these systems, where the focus is on the applicability and convenience of AI, often neglecting the major medium-term problem of the quality of its databases.
The next big struggle could be the decision to maintain blind trust in the outcome of these systems or to follow, for example, the path of filtering the information and data that AI works with.
The decision should depend on the purpose behind the use of AI. If that is, in a purely playful and exploratory context, the interest may be in the unpredictability of the result and not in its rigor and quality of the data source. In a work context, this lack of outcome control and system quality can call into question the credibility of its purpose.
When "human” data ends and the machines rely on data generated by themselves - the Full Circle - what will be the next steps for these systems in a business context?
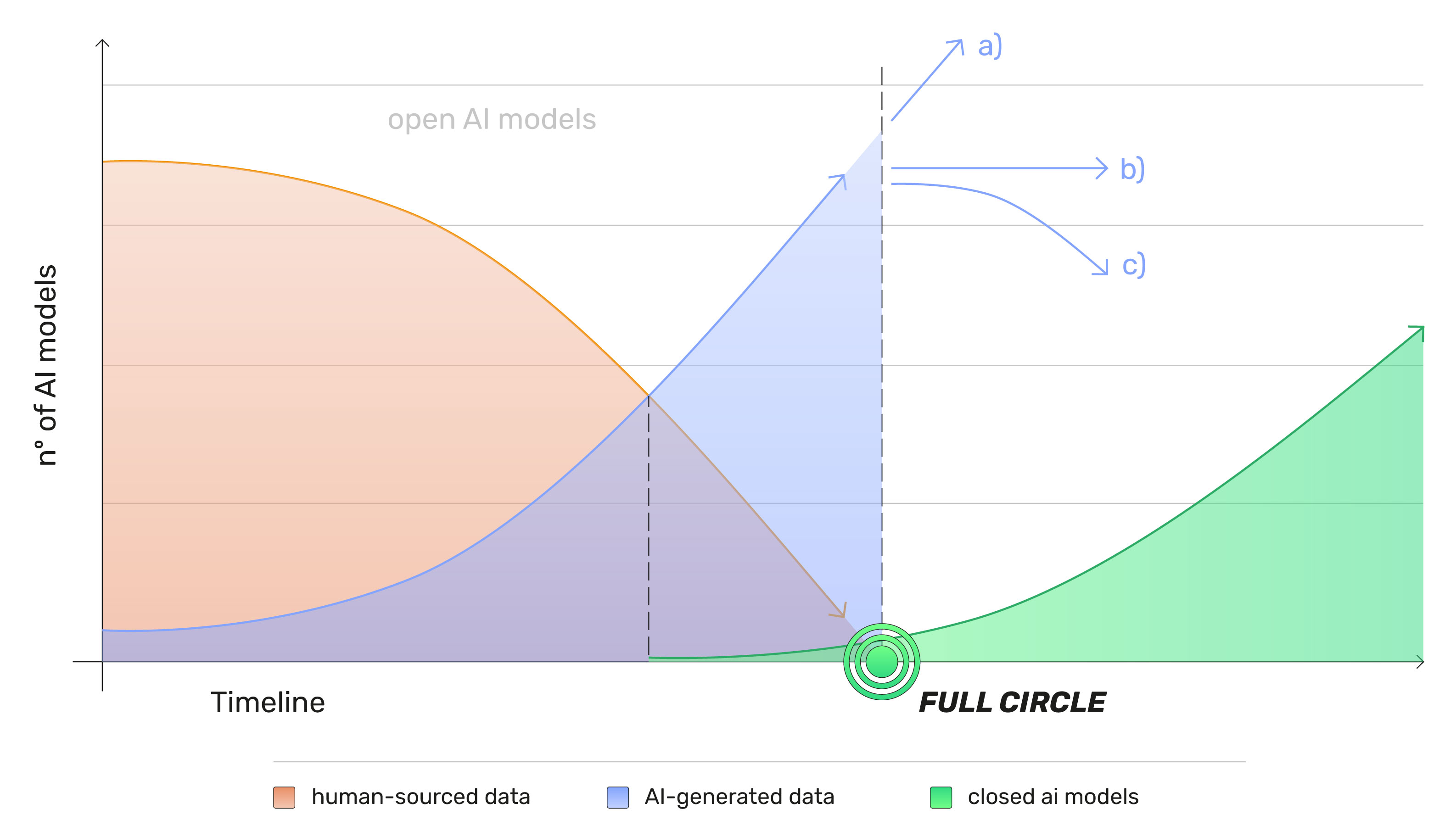
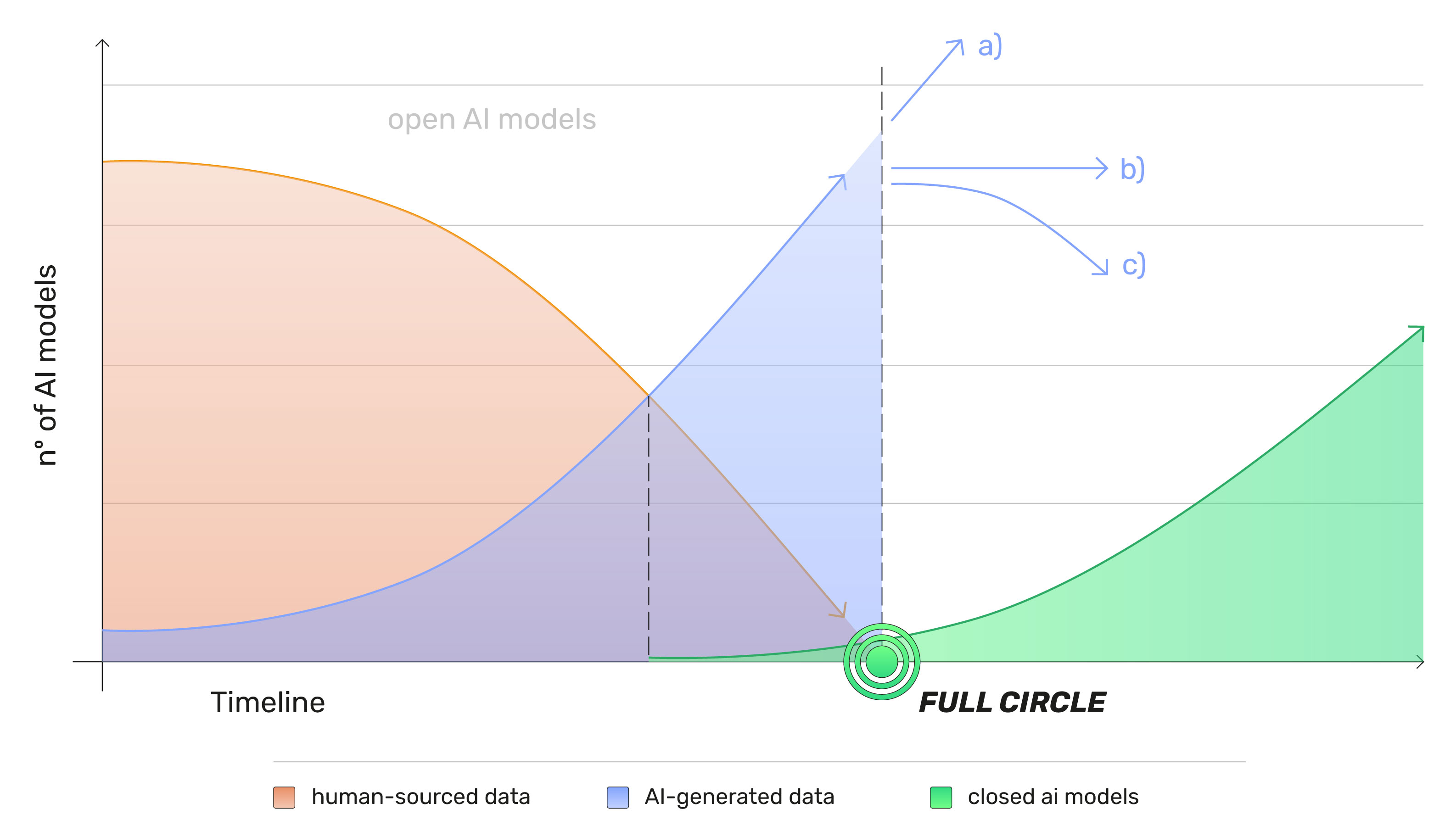
In my understanding, there are three possible approaches to the Full Circle phase, the alternative a) of continuation and blind trust in the outcome of the machine; the option b) of stagnation, which provides a period of consideration, with the analysis and tracking of the data from the systems (quality control); and the alternative c) with the decrease in the use of this type of (open) models in favor of limited systems and consequently controlled by the personalization of the database - closed AI models.
a) BLIND TRUST IN THE OUTCOME OF THE MACHINE
This alternative exposes the "hypocrisy” of the need for quality data, as for the exposure to "bad” data, this has always existed, whether in an open AI system or in the results of a common search engine. The Full Circle is not seen as a threat, it is just a new Era with data from a different origin (now generated by the machine), and it is not up to the receiver to define the quality of the outcome based on the criteria of the quality of the source data, but rather according to the correspondence to the expectation.
b) STAGNATION OR IMPASSE
The weighting period provides for the analysis and tracking of the data quality in the system. It is the alternative that presents the greatest challenges from a moral point of view, considering that such an assessment will have to be made by an individual or by a machine (involving a second system).
The problem with the individual is, firstly, that the feasibility of the task, how realistic will it be to manually track the data, and secondly, the assumption that an individual with specific training and limited knowledge surpasses the knowledge of a database (data from an open system) with the extra factor of the margin of human error. Making this scenario an unlikely option.
Regarding the machine scenario, if the origin of the problem lies in the trust and ability to evaluate the results coming from one machine, how will it be possible to trust the results of a second machine made to evaluate that same exact problem?
"We don't get out of the machine loop” – Bernardo Nunes (UX/UI Designer at OutSystems)
c) DECREASE AND ADAPTATION
The term decrease refers to the need to reduce popularized (open) AI systems, this alternative proposes the conversion of these systems to closed AI models. New models tailored to company’s data and objectives, creating a personalized and controlled system.
"Reliability is the precondition for trust” – Wolfgang Schauble
The next big challenge for AI could be the data quality processing and the ability of companies to develop AI models with longevity and flexibility.
Please note: the articles on this blog may not convey the opinion of .PT, but of its author.
Back to Posts